Constant Ecology Q&A
Chloé Hazelwood & Amalia Lindo
Chloé Hazelwood interviews Amalia Lindo about her Constant Ecology residency project
Q: What motivated you to develop a project for the Constant Ecology residency?
I had planned to travel overseas this year for three months to participate in two residencies. The first was in the Atacama Desert in Chile and the second in Mexico City, Mexico. Soon after the residencies were officially cancelled I heard about the Constant Ecology initiative. The Constant Ecology residency presented an opportunity to experiment with a recent idea that had developed alongside my PhD research. As my plans for the year had drastically changed, it was exciting to consider delivering this project in the context of a residency. I had never been a part of a residency program before and felt that Constant Ecology would offer a new platform for discussion around not just my own practice but equally, the work of other artists, writers and curators.
Q. Your project engages ‘crowdworkers’ via Amazon’s Mechanical Turk (MTurk) platform, paying them a fair wage for their involvement. What are some of the ethical issues around MTurk?
A few years ago I signed up to be a crowdworker on the Amazon Mechanical Turk platform. The title Mechanical Turk references an eighteenth-century chess-playing automaton of the same name designed by Wolfgang Von Kempelen. Kempelen’s Mechanical Turk appeared to exhibit human-level intelligence for the game of chess; however, the automaton was later revealed to be operated by a human concealed in a small cabinet beneath the chess board.
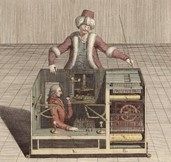
Amazon’s Mechanical Turk platform restages this illusion by asking human workers to complete HITs (human intelligence tasks) under the guise of machine-learning algorithms. As a worker, it was quickly evident that the working conditions on Amazon’s Mechanical Turk platform were of equal measure to the human uncomfortably folded beneath Kemplen’s automaton. The majority of HITs posted to Mechanical Turk offer a payment of 10 cents or less, allowing companies to use human workers to quality control the data produced by algorithms at minimal expense. By outsourcing this labour companies are able to pay a fraction of what it would cost for their employees to complete these menial, yet important tasks. The tasks themselves are simple, ranging from identifying cars in a blurry street image to transcribing data from receipts. In my experience as a Mechanical Turk worker, I was rarely paid more than 5 cents per HIT and it was common for my work to be rejected without explanation. As I started thinking through the logistics of this project an important component, for me, was for each worker involved in my project to be paid appropriately for their time and skills.
Q: What is the ‘task’ that you’ll have participants perform?
In my film practice, I have been working toward a series of video works that are produced using collections of found footage from the YouTube platform. The videos are collated according to specific sets of rules or patterns and are made in collaboration with algorithms designed with data scientist Dr. Timothy Lynam. I have used this formula over the last two years and have spent a considerable amount of time observing the daily activities of people from all over the world. I have wanted to expand my process so that I can begin to actively include the people who provide the video content used in my work. What interested me about Mechanical Turk were the similarities it shared with YouTube, as a platform hosting people from diverse social and cultural groups.
When the pandemic was declared and people around the world started working from home, this project felt particularly urgent as I wanted to provide workers with a task that not only paid a fair wage but was also engaging and asked them to share an experience from their own life. The current task asks workers to share videos taken from their mobile device that they found to be interesting or memorable. In addition, I have asked each worker to describe their video submission in a short sentence and to provide feedback on the task itself. Both the video and written components of the project have provided a fascinating glimpse into the lives of the workers behind Mechanical Turk. Going forward, I plan to experiment further with my task requests as a way to begin to align this project more closely with the methods applied in my wider practice.
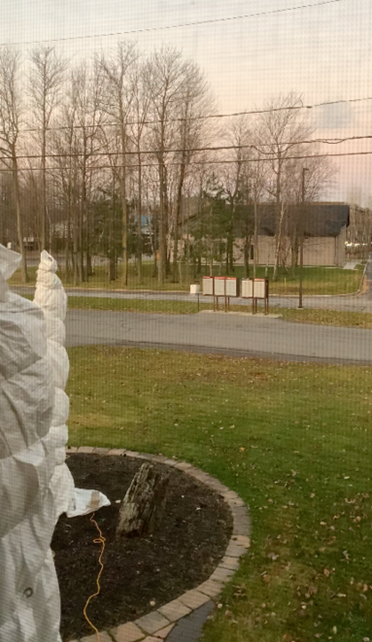
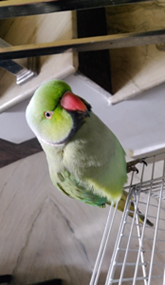

Q: What patterns or similarities have emerged in the data you collected on Mechanical Turk so far, and how might you collate the results into an eventual video work of your own?
I decided to begin the project using the task earlier described as I was curious to see how workers would respond to such an open-ended request. By asking for their (workers) own interpretation and subjective response to the task, there can really be no wrong answer. The task feedback provided by some workers has indicated how rare it is for the outcome of a task to reflect the individual who has completed it.
To date, I have received submissions from North America, Canada, United Kingdom, Greece, United Arab Emirates, Thailand, Brazil, Argentina, India and Thailand. A common denominator in the videos submitted so far has been house pets, with many workers commenting that their animals have been their only companion in a year of continued isolation. Other common features have been views from apartment windows and contents of home offices or bedrooms. The similarities presented by the video results and written descriptions has been most interesting to observe, not only for the content itself but for the videography too. For me, this is the beginning of an expansive experiment with Mechanical Turk, one with the potential to develop into a layered video installation. I am still deciding where the narrative thread of the project will lead, but I intend to find a way to weave my own algorithms back into the process of editing to see what results are produced. For now, stay tuned.
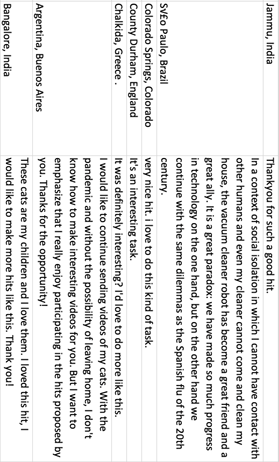
Q: Your research involves the study of algorithms, and you’ve worked with a data scientist on the design of this project and in your previous work. What have been your findings in terms of how humans and algorithms differently interpret visual data?
My PhD research extends the investigations of my video and installation practice, which I briefly described earlier. I have been working together with Tim Lynam to develop a number of algorithms capable of replicating certain ‘creative’ methods applied in my filmmaking process. The first algorithm is applied in the process of video data collection, and the second algorithm is then programmed to self-produce a video from the data collected using certain pre-defined rules. To put it simply, together the algorithms collect, edit and produce a film. To teach the algorithm how to achieve this I attempted to translate not only my editing techniques, but also the conceptual analysis underpinning my editing process. I found this to be, of course, complicated. So much of the joy of filmmaking, for me, comes from the unexpected collisions that emerge in combining footage. While the algorithms have certainly produced interesting results, there is a level of visual complexity that seems to have been lost in translation. This isn’t to say that the algorithms’ results are not complex, or that the discrepancies I have observed are not the fault of its designers. I think what has been interesting about this experiment has been witnessing how and where the challenges I have observed are reflected in wider discussions around image recognition technologies and algorithmic bias.
Chloe Hazelwood is the current Chair of the Blindside Board. She is a curator, arts writer and arts manager living and working on the traditional lands of the Wurundjeri people of the Kulin Nation.
Amalia Lindi is an artist based in Melbourn. Lindo’s moving-image and installation practice considers the evolving interrelations between humans and artificial intelligence.